The impact of AI on the cost of starting and running a business
DDVC #33: Where venture capital and data intersect. Every week.
👋 Hi, I’m Andre and welcome to my weekly newsletter, Data-driven VC. Every Thursday I cover hands-on insights into data-driven innovation in venture capital and connect the dots between the latest research, reviews of novel tools and datasets, deep dives into various VC tech stacks, interviews with experts and the implications for all stakeholders. Follow along to understand how data-driven approaches change the game, why it matters, and what it means for you.
Current subscribers: 7,947+, +200 since last week
Brought to you by Gravity - Your all-in-one platform with billions of data points on founders and startups
Unlike other data providers, Gravity tracks new company launches and all funding events each month while diving deep into the key insights around the founders of these companies in an easy-to-use dashboard that helps you uncover their FounderDNA™ - founder characteristics such as prior exits, past experiences and much more! Sign up for free and take advantage of the 14 day free trial
Every board meeting in 2023 thus far had the same item on the agenda: What’s the impact of AI on our business? More specifically:
What’s the impact of AI on our own product?
What’s the impact of AI on our daily routines and workflows?
Today, I’d like to share my generalized perspective on both of the above questions and why I believe it will be significantly easier to start a software company in the future. Please challenge my thoughts and share your perspectives with me!
1) The impact of AI on your product
Of course, the answer to this question depends a lot on the product. Flipping things around and looking at the question from a user’s perspective, there are two main groups of companies/products:
Existing (non-AI) incumbents like Microsoft (with “AI-powered Teams Premium”), Notion (with “Notion AI”) or Duolingo (with “Duolingo Max”) extending their well-established and broadly adopted product offering with AI-powered features.
New (AI-native) challengers like Jasper, CopyAI or Runway rethinking products from scratch with AI in their core.
While the “non-AI incumbents” are likely to have great penetration and large, trusted user bases that are familiar with their products, “AI-native challengers” are able to leverage this new technology and rethink their solutions from scratch. Wearing my investor hat, the major question is whether add-ons can be as good as something purposefully designed from scratch?
Clearly, challengers believe the answer is no. This is why they started their companies in the first place. The majority of companies across startups, scale-ups, SMBs and enterprises, however, falls into category 1 above. Therefore, they should urgently explore how AI-powered add-ons or even new product lines can bring their offerings as close to AI-native solutions as possible.
The longer incumbents wait to add AI-powered features, the higher the chances their customers see their own competitors getting more done with less by leveraging other AI-native solutions. At some point, FOMO will exceed the friction and switching costs of migrating to a new AI-native tool, resulting in slowly but gradually fading customer bases for the incumbents.
Take a user-centric view to explore potential for AI-powered features in your existing product
Depending on the stage of the company, products are more or less complex. The less (more) established the company and product, the easier (more difficult) it becomes to change fundamental parts of the product.
Independent of the existing product complexity, it seems most intuitive to take a user-centric view, map all of the workflows that can be executed with the existing product (shadowing users helps a lot), and then scrutinize every single step to see if and how it can be automated/changed with LLMs.
Here, incumbents can benefit from external expertise as well as partnerships and deep integrations with LLMs providers. At some point, they might even want to consider verticalized LLMs tailored to their business, just like Bloomberg GPT.
2) The impact of AI on your workforce
While Morgan Stanley and others predict AI to create a $ 6+ Trillion opportunity and significant productivity gains to be captured, the majority of desk workers haven’t changed their workflows or tool stack at all. Likely, because incumbents are just starting to launch AI-powered features and the fact that most professionals didn’t yet face enough pressure to leave their comfort zone and test out AI-native solutions. This will change. Soon.
As a result, I expect every knowledge worker, literally everyone who performs a task in front of a screen, to leverage AI and create more as well as better output with less input. It started with developers (👋🏻 Github Copilot), continued with communication (👋🏻 Jasper) and designers (👋🏻 Stability), and will soon be prevalent across sales, customer support, HR and every other part of a company.
Our findings reveal that around 80% of the U.S. workforce could have at least 10% of their work tasks affected by the introduction of LLMs, while approximately 19% of workers may see at least 50% of their tasks impacted. - Working paper published March 27, 2023 by OpenAI and University of Pennsylvania
Clearly, companies will get more done with less resources. This will have two implications. Firstly, considering a fixed target output (users, revenue, growth etc.), the input and required resources can be reduced. This would mean less hiring, less replacements and more lay-offs to reduce the workforce. Secondly, considering a fixed input (workforce), the output can be increased. In this scenario, it’s questionable whether there will be natural limits to growth. In any case, both scenarios mean more efficient operations.
Zooming out: What does this mean for new startups?
During my PhD days at the Technical University of Munich, one of my colleagues researched the impact of different technologies on the “cost of experimentation”, a term to describe the resources required to start a business. A well-cited study in this domain is the “Cost of experimentation and the evolution of venture capital” paper by Ewens, Nanda, and Rhodes-Kropf (2018).
The introduction of cloud computing services by Amazon is seen by many practitioners as a defining moment that dramatically lowered the initial cost of starting Internet and web-based startups. (..) We show that subsequent to the shock, startups founded in sectors benefiting most from the introduction of AWS raised significantly smaller amounts in their first round of VC financing. (Ewens, Nanda, and Rhodes-Kropf, 2018)
The conversations in our different board meetings made me think of this paper. Will the widespread adoption of AI be the second “cloud moment”?
My answer is yes. I expect that the cost of experimentation will significantly drop (again), probably even more than it did after the introduction of cloud computing. Next-gen founders will be able to set up their companies as one (wo)man show and the help of specialized autonomous agents like AutoGPT or BabyAGI.
For many founders, little additional resources will be required to test a market hypothesis and build a huge company on their own.
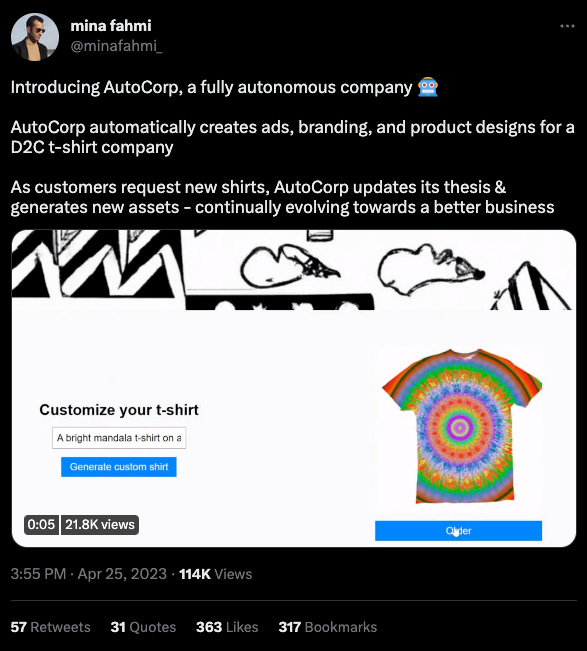
But what happens once product-market-fit got validated? Well, in this context, we need to think about the “cost of running the business at scale”.
The primary effect that AWS had was on the initial fundraising by startups rather than on the cost of running the business at scale — effectively allowing startups to shift their large capital investments to later stages when uncertainty had been resolved. (Ewens, Nanda, and Rhodes-Kropf, 2018)
While certainly the costs of running a business at scale will be reduced through above-described AI-powered productivity gains, I believe that the impact will be relatively higher on the cost of experimentation and the initial stages of a business.
Setting up an MVP will become possible with very little resources, whereas it requires experts to take thoughtful decisions and significant resources to scale a global company beyond product-market-fit. 80/20 is sufficient in the beginning, but building a category defining/leading company requires 100% outperformance of the market. I expect that human experts will continue to have significant impact here, and these few individuals will likely become even more expensive in the labor market.
What does this mean for investors?
Effectively, the question is whether the number of milestones to be achieved with a fixed amount of capital will increase (=more runway) or whether the resources required to achieve a fixed milestone will decrease (=smaller rounds).
Intuitively, I’d say that Pre-Seed and Seed round sizes are likely to decrease as more capital does not necessarily increase the chances of achieving product-market fit. This would also mean that funds dedicated to these stages would eventually become smaller.
In line with my arguments above, I expect a different dynamic for the growth stages where it’s a lot about excellence and execution. For Series A+, it’s likely that round and fund sizes will stay unchanged. I’d expect that companies will either grow more profitably and extend their runway or parallelize activities to dominate the market even more aggressively.
Exciting times ahead.
Stay driven,
Andre
PS: Check out the “Riding Unicorns” podcast by my friends James and Hector to explore the ins and outs of tech unicorns with some of the leading VCs and operators
Thank you for reading. If you liked it, share it with your friends, colleagues and everyone interested in data-driven innovation. Subscribe below and follow me on LinkedIn or Twitter to never miss data-driven VC updates again.
What do you think about my weekly Newsletter? Love it | It's great | Good | Okay-ish | Stop it
If you have any suggestions, want me to feature an article, research, your tech stack or list a job, hit me up! I would love to include it in my next edition😎